Data is king when it comes to developing new machine learning algorithms. So earlier this month SciTec conducted a field test to collect infrared and visible data on representative drone swarms to support development of new algorithms for the counter-small unmanned aerial system (sUAS) mission.
The threat of adversary or criminal use of sUAS against our military forces, critical infrastructure, and populace is a growing issue. To counter these threats, we first need to detect them, and preferably with automated systems. That’s where artificial intelligence and machine learning (AI/ML) comes in.
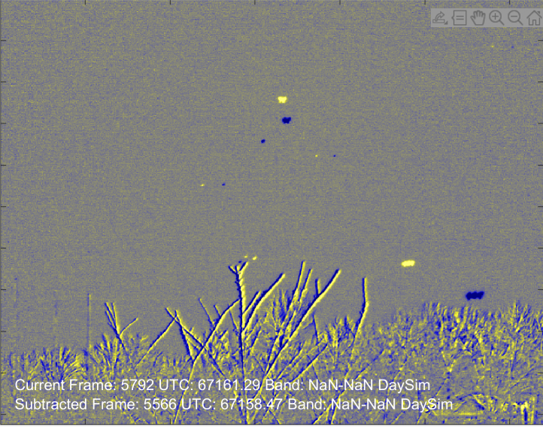
“SciTec has an excellent reputation in developing AI/ML algorithms for missile warning and defense missions. We believe these same algorithms can be applied to the counter-UAS mission” said Damien Turchi, SciTec AI/ML Scientist and Principal Investigator for this field test. “We just needed to collect enough data on a variety of representative UAS threats with a number of different cameras to get started on training the algorithms. We executed this field test under our independent research and development (IR&D) program.”
The swarm scenarios featured up to nine sUAS ranging from a small custom racer to a commercial grade hexacopter. Pilots from the Drone Academy in Ringoes, New Jersey and SciTec, flew pre-planned patterns designed to represent swarming activity. Other SciTec operators supported data collection from a variety of commercial shortwave, midwave and longwave infrared (SWIR, MWIR, LWIR) and visible cameras. Preliminary data show great results with both point- and extended-source targets, multiple targets in a scene, and enough background clutter and confusers to provide real-world processing challenges.
“SciTec is engaging with a number of industry partners and Government agencies on the counter-UAS mission,” stated Craig Falci, Lead Portfolio Engineer. “This field test collection provides us unique data on heterogenous swarms to further develop our detection and tracking solutions.”
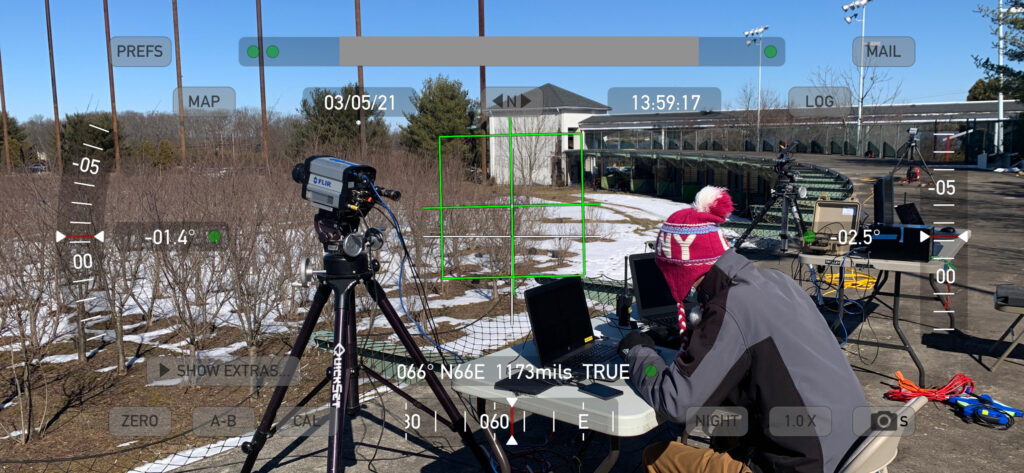
Damien added, “Our frame processing software handled the imagery data right out of the box. We’ll continue our IR&D effort to tune and train our algorithms to provide a demonstrable threat warning capability we can deliver as part of a future integrated solution.”